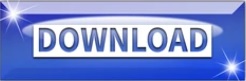
A number of these processes remain outside the capability of standard statistical procedures, requiring equally complex computational models in order to more accurately reflect physical relationships. Many complex system interactions are present throughout the disciplines of geophysics and associated solar dynamics. Keywords:Space Weather, Geomagnetic Storms, Artificial Neural Networks, Solar Winds, NARX, Forecasting, Interplanetary Magnetic Field

Furthermore, it is found that a Bayesian regularization neural network algorithm may be the most accurate model compared to both other forms of artificial neural network used and the linear models employing regression analyses. By assessing various statistical techniques it is determined that artificial neural networks may be ideal for the prediction of disturbance storm time index values which may in turn be used to forecast geomagnetic storms. Given the occurrence of solar activity hours or days before subsequent geomagnetic fluctuations and the potential effects that geomagnetic storms have on terrestrial systems, it would be useful to be able to predict geophysical parameters in advance using both historical disturbance storm time indices and external input of solar winds and the interplanetary magnetic field. This ring current produces a magnetic field which opposes the planetary geomagnetic field. Received 31 January 2014 revised 28 February 2014 accepted 18 March 2014Ī nonlinear autoregressive approach with exogenous input is used as a novel method for statistical forecasting of the disturbance storm time index, a measure of space weather related to the ring current which surrounds the Earth, and fluctuations in disturbance storm time field strength as a result of incoming solar particles.

#Omniweb nasa license
This work is licensed under the Creative Commons Attribution International License (CC BY). Neuroscience Research Group, Laurentian University, Sudbury, CanadaĮmail: © 2014 by author and Scientific Research Publishing Inc.
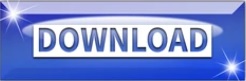